Keynote 1 – November 9, 5.30pm-6.30pm Milan, 11.30am-12.30am NYC
Carbon Aware Computing at Google
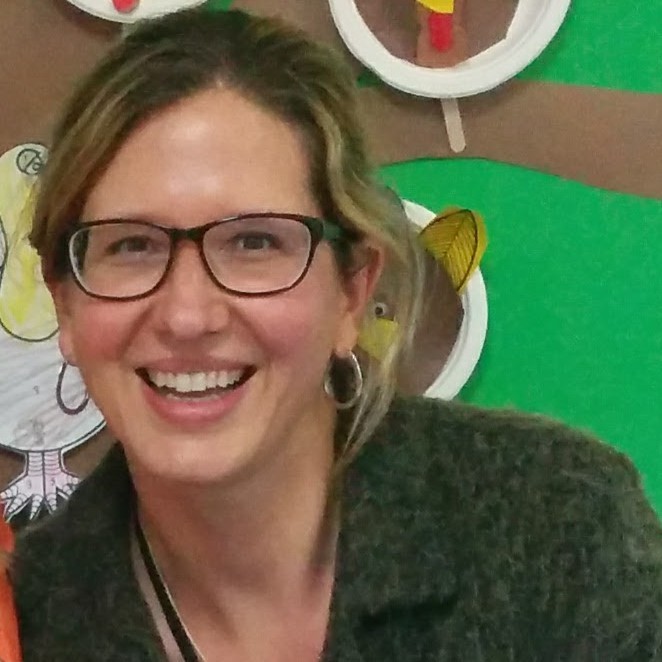
Ana Radovanovic has been a research scientist at Google since early 2008, after she earned her PhD Degree in Electrical Engineering from Columbia University (2005) and worked for 3 years as a Research Staff Member in the Mathematical Sciences Department at IBM TJ Watson Research Center. For the last 8 years, Ana Radovanovic has focused all her research efforts at Google on building innovative technologies and business models with two goals in mind: (i) to deliver more reliable, affordable and clean electricity to everyone in the world, and (ii) to help Google become a thought leader in decarbonizing the electricity grid. Nowadays, Ana is widely recognized as a technical lead and research entrepreneur. She is a Senior Staff Research Scientist, serving as a Technical Lead for Energy Analytics and Carbon Aware Computing at Google.
Abstract
The growing climate impact of increased Greenhouse Gas Emissions and CO2 levels in Earth’s atmosphere highlights the value and importance of technologies that reduce such impact. Electricity generation is a significant contributor to global CO2 emissions, and the datacenter industry is expected to reach anywhere from 3 to 13% of global electricity demand by 2030. Datacenters have the potential to facilitate grid decarbonization in a manner different from isolated power loads, and Google has set on a mission to increase and scale carbon awareness via new technology solutions. We present Google’s globally-distributed Carbon-Intelligent Computing System, which harnesses datacenter load flexibility, not only to reduce emissions, but also to contribute to more robust, resilient, and cost-efficient decarbonization of the grid through energy system integration. We share some conclusions derived from operational data and discuss the key technical challenges that drive carbon-aware designs and their future enhancements. By calling out opportunities for “greening” the cloud industry, we hope to inspire academia and industry to pursue diverse and system-specific carbon-aware solutions.
Keynote 2 – November 10, 5.30pm-6.30pm Milan, 11.30am-12.30am NYC
Collective decision-making
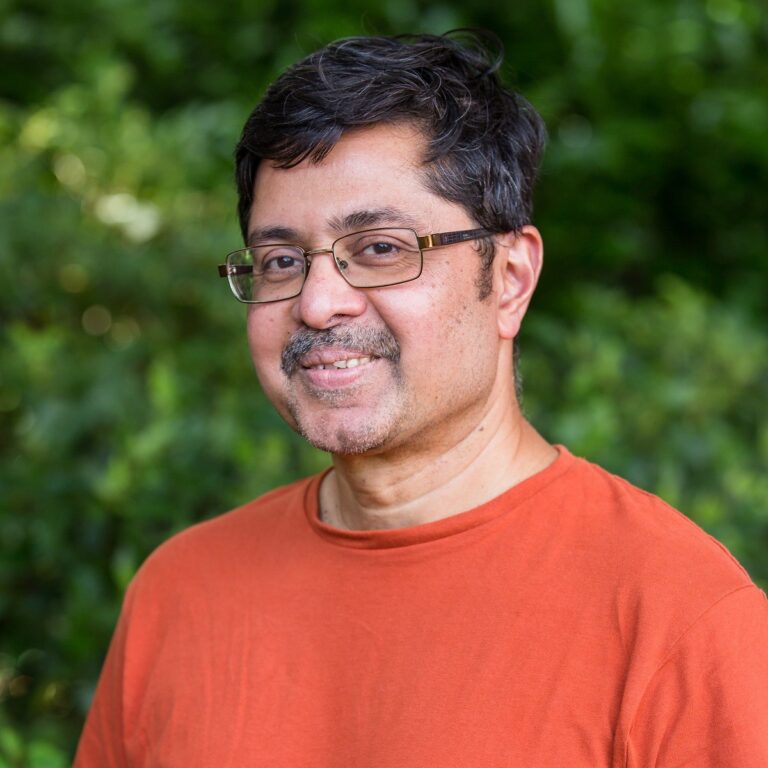
Ayalvadi Ganesh is an Associate Professor at the School of Mathematics, University of Bristol. His research interests include large deviations, queueing theory, random graph dynamics, and decentralised algorithms. He won the INFORMS Best Publication Award in 2005 and the ACM Sigmetrics Best Paper Prize in 2010. He is co-author of the monograph, Big Queues.
Abstract
The problem of selecting the best option amongst several is an everyday occurrence, not only for human beings but also for other biological organisms and other collectives of autonomous agents. Typically, these decisions have to be made under uncertainty and imperfect information, and different agents may have different information about the options. The objective is to combine their information effectively so that the group as a whole can make the best decision. This often needs to be done with only limited communication permitted between agents. In this talk, we will discuss a few different formulations of this problem, for which we will present algorithms and performance bounds.
Keynote 3 – November 11, 5.30pm-6.30pm Milan, 11.30am-12.30am NYC
Database alignment: fundamental limits and efficient algorithms
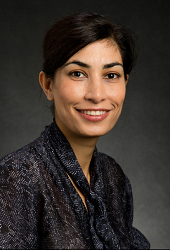
Negar Kiyavash is the chair of Business Analytics (BAN) at École polytechnique fédérale de Lausanne (EPFL) at the College of Management of Technology. Prior to joining EPFL, she was a faculty member at the University of Illinois, Urbana-Champaign, and at Georgia Institute of Technology. Her research interests are broadly in the area of statistical learning and applied probability with special focus on network inference and causality. She is a recipient of the NSF CAREER and AFOSR YIP awards.
Abstract
As data collection becomes ubiquitous, understanding the potential benefits as well as the risks posed by the availability of such large amount of data becomes more pressing. Identifying how data from different sources relate to each other, could allow to merge and augment data. On the positive side, this could help for instance in deducting functionality of proteins by comparing protein interaction networks of different species. On the negative side, such alignment could cause unintended exposure of confidential information. A famous case of such breach occurred when customer data from the anonymous Netflix Prize database was revealed through alignment with public IMDB profiles.
In this talk we present information-theoretic results on database alignment, showing how the size of databases and the correlation between their elements determines the success of alignment. Database alignment is closely related to equally interesting problem of network alignment, a generalization of the graph isomorphism problem.